In our recent, Global Pricing Study we established that 40 to 60% of innovations fail to meet financial targets or fail entirely. We also find mixed signals about profitability of highly innovate AI companies (e.g., Anthropic’s 50-55% margin), which shows that a point raised by Andreessen Horrowitz in 2020 that AI gross margins are often 10-20 p.p. lower than SaaS peers remains relevant.
As SaaS companies strive to innovate and deliver more value to their customers, the integration of Generative Artificial Intelligence (Gen. AI) features presents a unique opportunity. By harnessing the power of Gen. AI, the technology provides unique opportunities to unlock revenue growth. In our recent Global Software Study, 73% of SaaS executives expect Generative AI to lead to more than 10% increase in revenue. Yet, for many companies there is no clear plan on how to realize this. Given all we know about lower margins and pitfalls for new innovations, how can SaaS companies create a sustainable business?
We know from our experience working with SaaS and AI-native companies that a good monetization strategy is key. In our previous article, we discussed considerations between giving the added value of Gen. AI features away for free (anticipating future gains) versus pricing these features and monetizing them directly. This article dives deeper into the world of direct monetization and provides six practical packaging and pricing best-practices for Generative AI.
Best practice 1: Understand the feature’s role in the packaging line-up
The foundation of a successful monetization strategy begins with a comprehensive understanding of the feature’s role within the product. For this, two questions are relevant: “1) How many (prospective) customers would use this feature?” and “2) To what extent would customers that use this feature be ready to pay a large (or small) price premium?”. By answering these questions, businesses can assess the feature’s potential to drive revenue and categorize it as a leader (high interest and willingness to pay), filler (high interest, but low willingness to pay), add-on (low interest, but high willingness to pay), or killer (low interest and low willingness to pay).
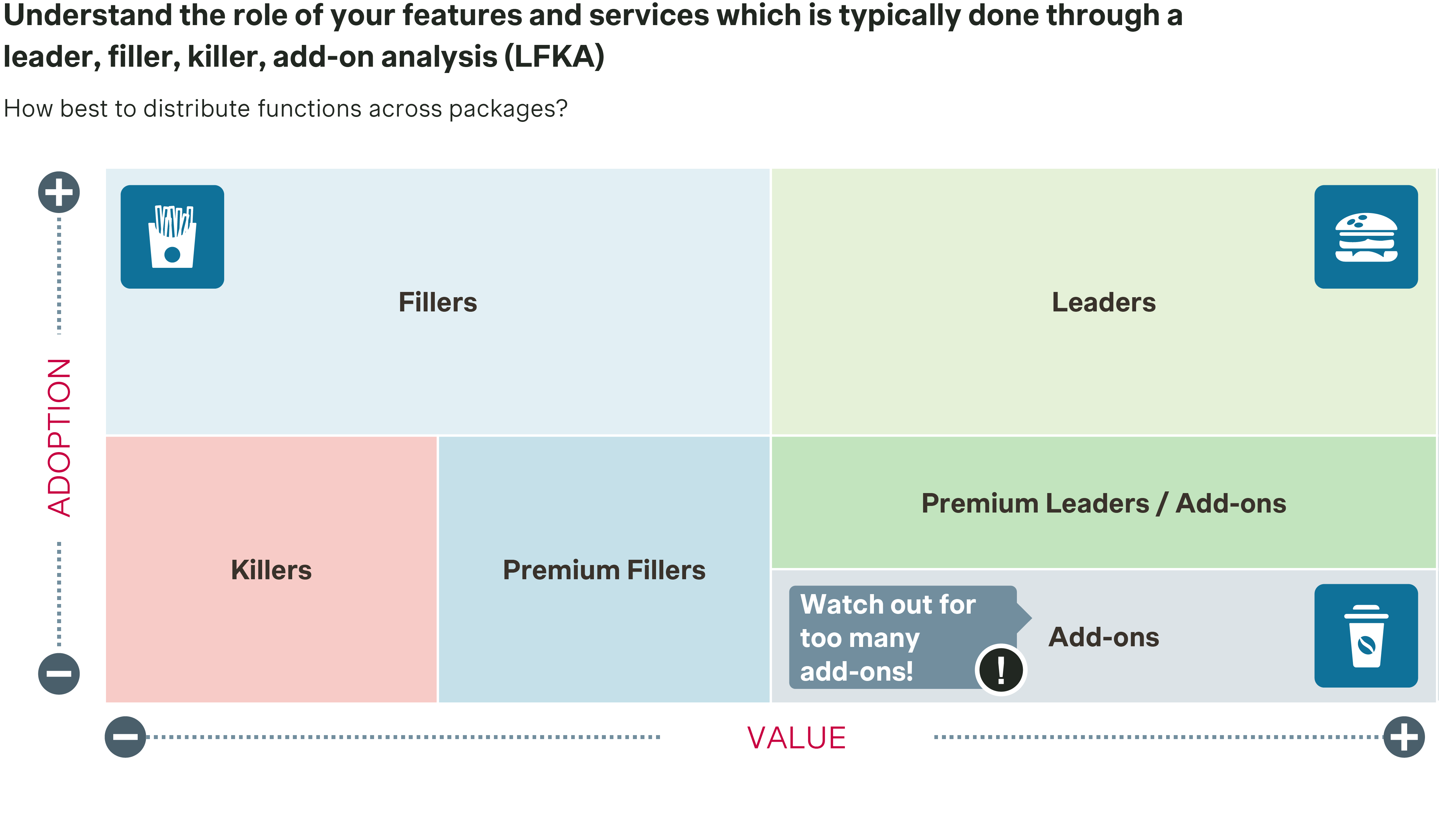
We find that approximately 50% of SaaS companies have a Good/Better/Best packaging structure and in our projects we often see that Gen. AI features are leader features. When multiple Generative AI features can be categorized as leader or filler, it is best to divide them across the Good-Better-Best packaging to create an attractive up-sell path. Furthermore, we recommend allocating the features based on what customer segment or use-case each package is meant to address. The outcome should be an attractive land and expand packaging structure with a clear and unique story for every package. GitHub Copilot follows this best-practice by having a clear use-case per package (one package that is tailored to individuals while offering two packages for organizations).
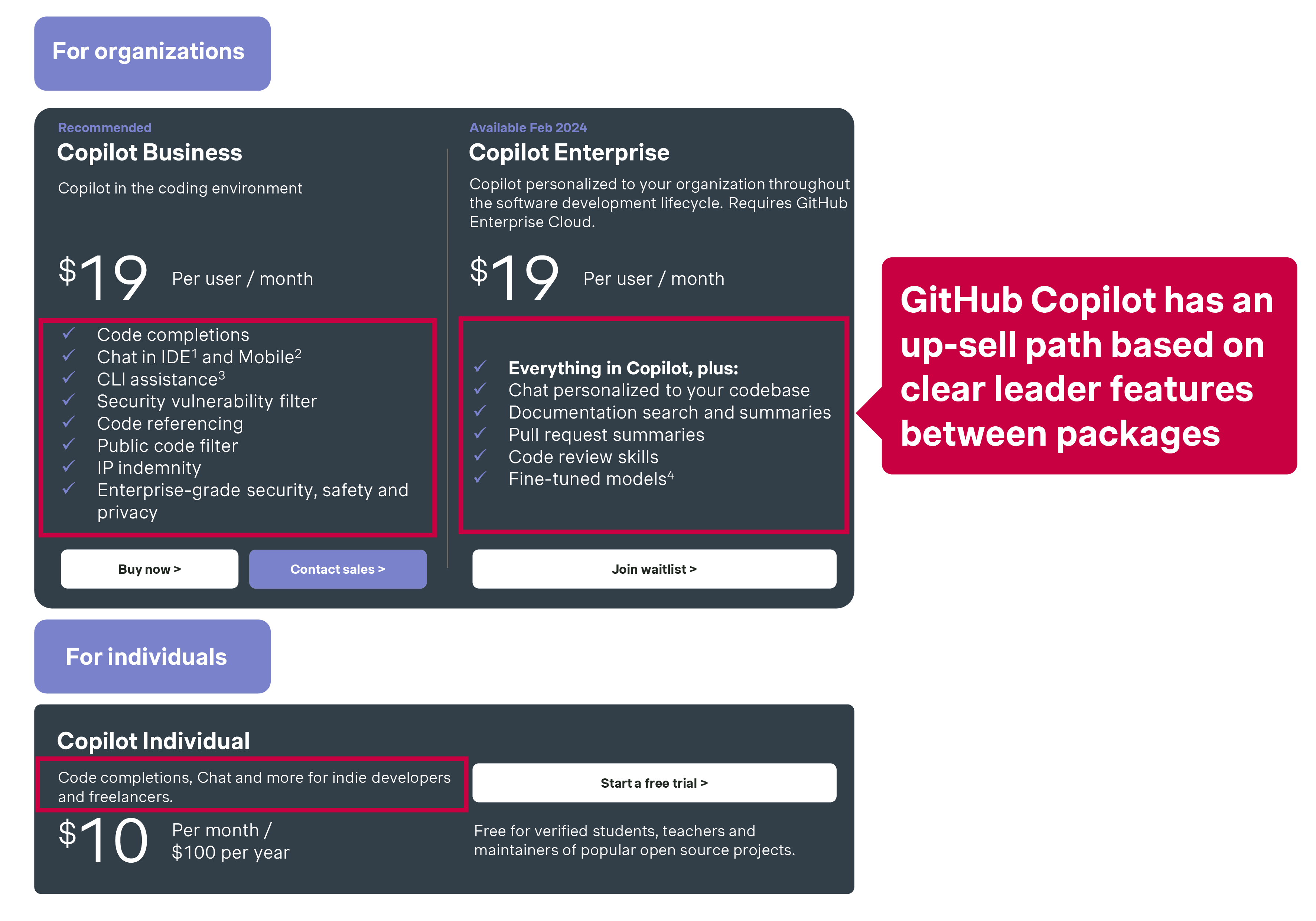
Example: GitHub Copilot (Code editor)
Best practice 2: Differentiate Generative AI features between packages
It is not necessary to have all Generative AI features just in a single package or add-on. There is in fact an opportunity to differentiate this between package to cater to a wider range of customer needs and budgets. For instance, by offering basic Generative AI functionalities (e.g., text processing) in the entry-level package while reserving more advanced features (e.g., image or video processing) in premium tiers. Similarly, the speed or accuracy of a model can be differentiated (e.g., GPT 3.5 in the entry tier vs. GPT 4.0 in a premium tier), which can create an up-sell path and save costs in entry-level subscriptions. We find that OpenAI’s ChatGPT follows this best-practice, which reportedly converted millions of subscribers to their paid tier with a better model and more features, while providing access to their less advanced model that cost them less in their lower tier. Writesonic, an AI-enabled text editor, is another example which does this well.
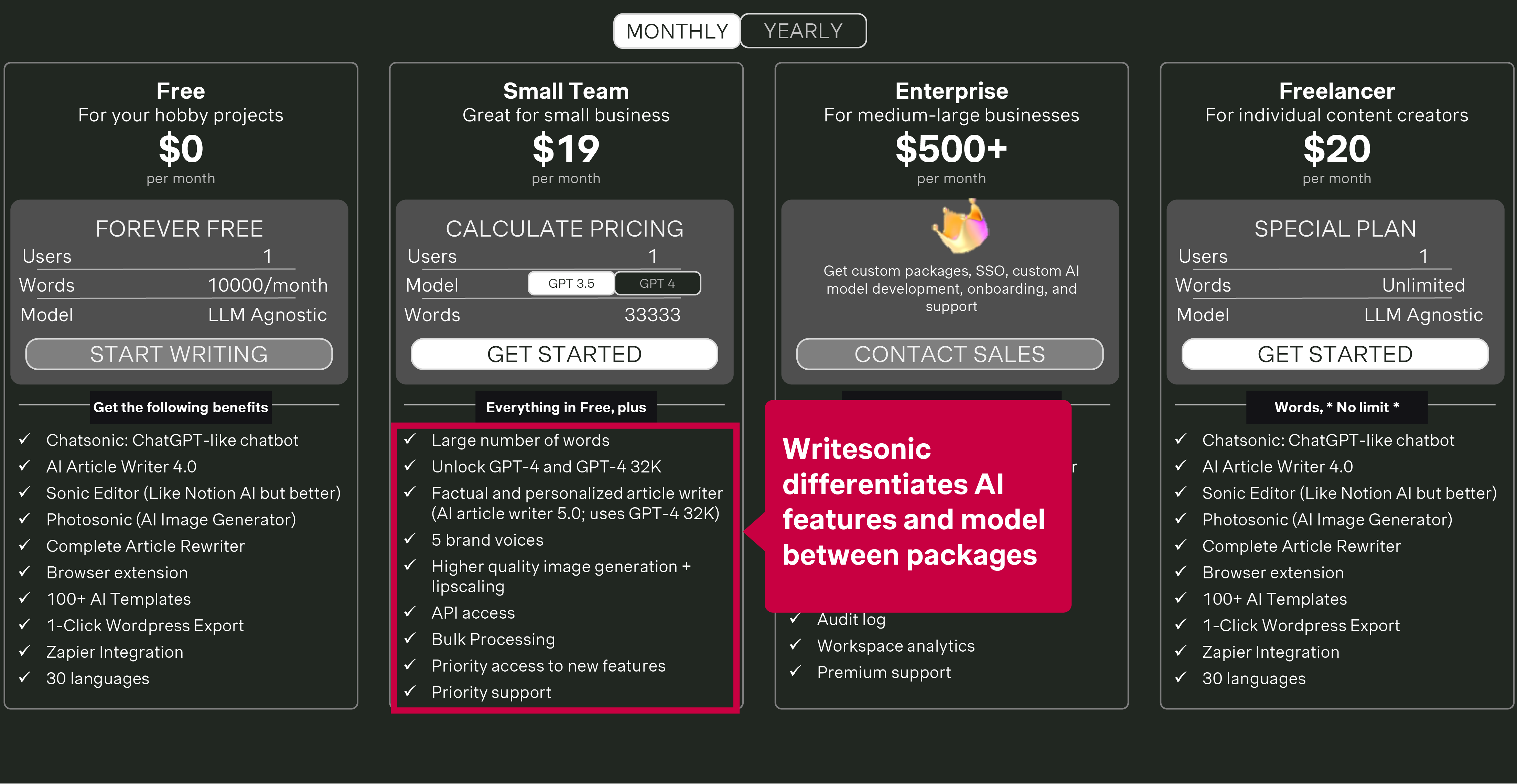
Example: Writesonic (Text editing tool)
Best practice 3: Select the right price metric
Although usage-based pricing has been a pricing trend across the SaaS industry in recent years, there is quite some variation for Generative AI solutions. Some have a user-based fee whereas others work with credits or a price per query. The ideal price metric adheres to the following principles: it should be straightforward to explain, it should scale with value, and it should be implementable while ensuring transparency and fairness in billing.
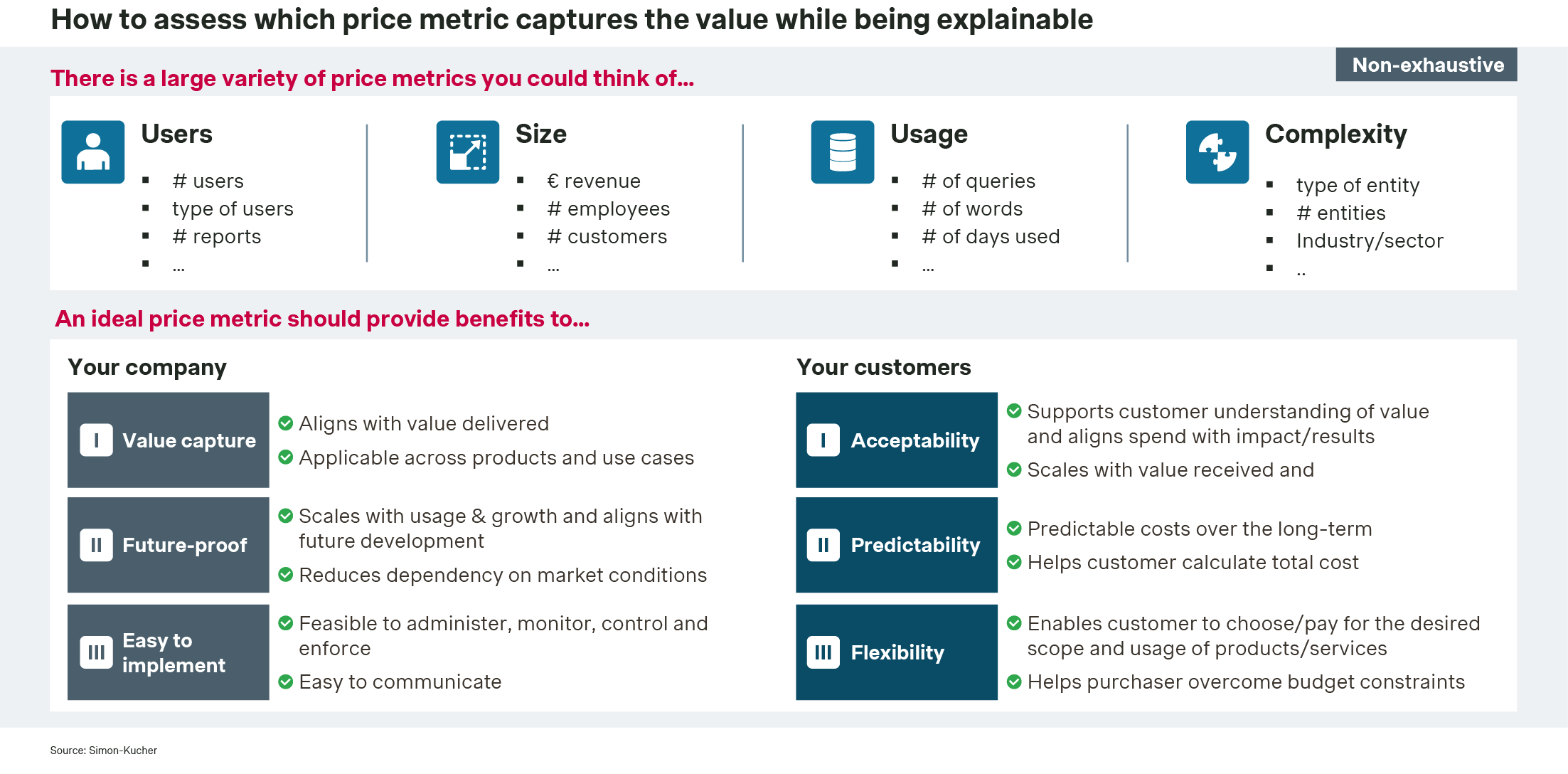
A user-based fee for Generative AI that guarantees fair usage and has fences in place to up-sell heavy users is often very explainable and easy to implement. Yet, we do recommend challenging yourself to understand (e.g., through customer research) whether other usage-based metrics can be a better fit. We’ve worked with clients where AI features were an immediate threat to the number of users given the productivity gains it provided, which shows that user-based fees are certainly not always optimal. Usage-based metrics are typically an excellent way to land and expand customers over time while scaling the price with the value which the customer experiences. Usage-based metrics can be used as fencing mechanisms between packages (e.g., offering up to X queries in package A and up to Y queries in package B) to avoid that customers feel like they are being charged for every single action.
Example: Adobe Firefly (Image generation tool)
Best practice 4: Implement a fair-use policy
Some Gen. AI applications choose to allow ‘unlimited’ usage. As such, they promote frequent and intense usage. This is how GitHub Co-pilot last year reportedly lost $20 a month per user, with some heavy users causing losses of $80 per month. To mitigate the risk of unexpected high usage leading to resource strains, which may result in either dissatisfied customers or disproportionately high costs without the corresponding monetization of value, it is advisable to establish a fair-use policy. This way clear usage boundaries are set while maintaining an open and honest relationship with customers. It also ensures the service remains sustainable for the provider and affordable for the user. Having reached the limit, we typically see that users are either refused access to the feature or can continue to generate content at significantly reduced speeds (with the latter being a more customer-friendly option).
Example: Fireflies.ai (Note taking tool)
Best practice 5: Determine the right price level
Setting the right price involves a good understanding of value to customers and, particularly for Gen. AI features, of costs. To set the right price level, we typically use a multimethod approach, including but not limited to customer research, competitor analysis, and cost analyses. Combining those perspectives helps to identify a price point that resonates with the target audience while sustaining a healthy profit.
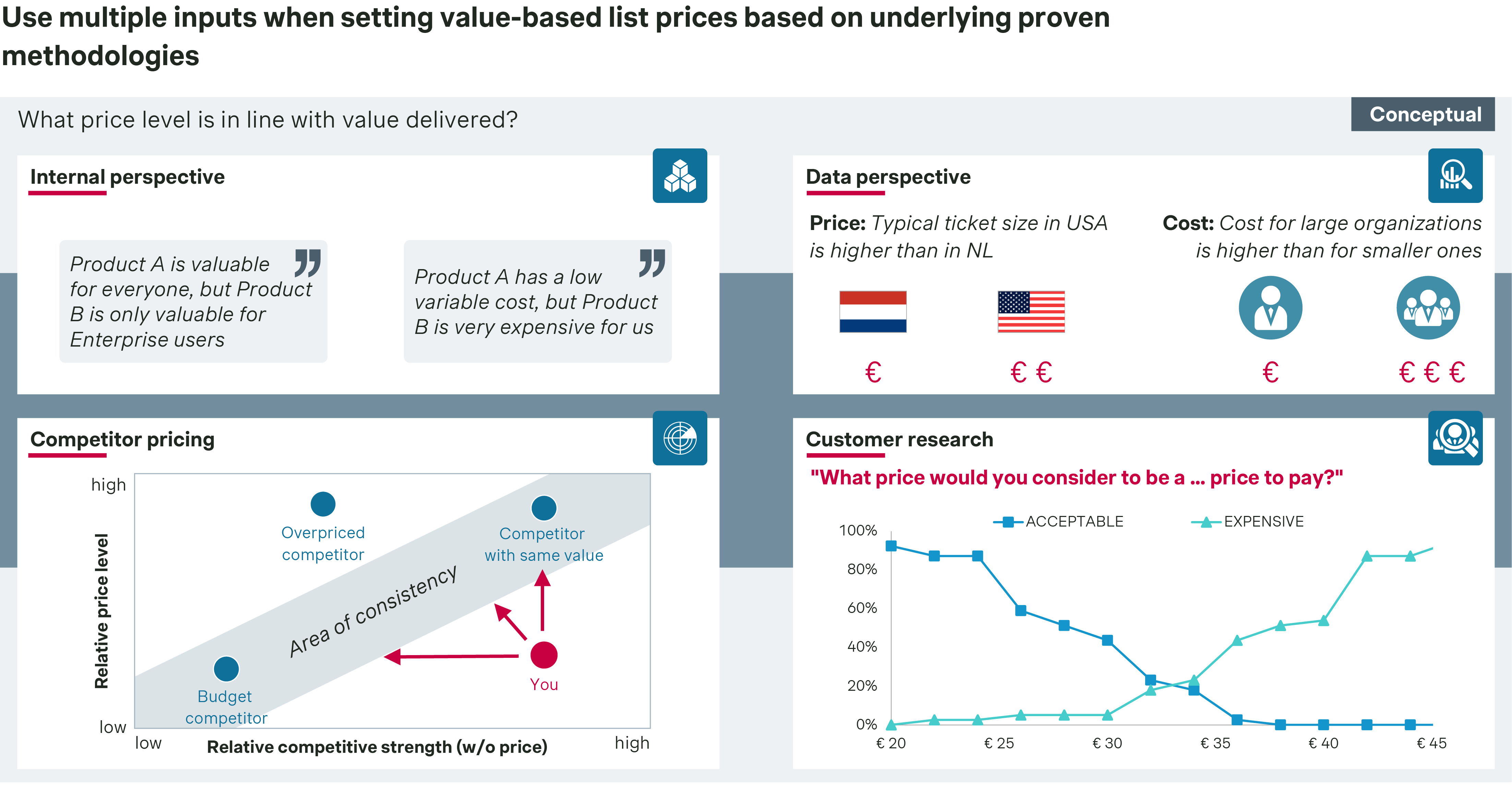
Last year, workflow solution provider ServiceNow launched its Generative AI-based “Plus” packages at a 60% price premium. ServiceNow had gathered feedback from 150+ customers on early versions to understand the value it delivers. At first the industry was skeptical of this seemingly hefty price premium, but in their 2023 Q4 earnings call ServiceNow CEO Bill McDermott reported the sales cycles of these packages to be shorter, adoption to be significantly faster and willingness to pay to be no issue: “There's a real appetite to invest in Gen AI, and there's no price sensitivity around it because the business cases are so unbelievable. I mean if you're improving productivity, 40-50%, it just sells itself.” If ServiceNow had charged a lower price it would have left a lot of money on the table.
Example: ServiceNow (Workflow management tool)
Best practice 6: Communicate value effectively
The significance of clear and simple communication cannot be overstated. Moving away from complex technical jargon towards easy-to-understand language helps in articulating the value of Generative AI features. By focusing on how the feature enhances the customer's operations or solves specific problems, businesses can increase the perceived value, encouraging greater adoption and willingness to pay. Likewise, package-specific value messaging can help to steer specific groups of customers to the subscription that is designed to best fit them. We find that ClickUp, a productivity tool, follows this best-practice by explaining the value of their AI features in simple words.
Example: ClickUp (Productivity tool)
In conclusion, adopting these six best practices when packaging and pricing Generative AI features is key to achieve better monetization results. Are you interested in how we can support you to make Generative AI a commercial success for your business? We can help you think about use cases to leverage the potential of Generative AI and we can provide a data-driven perspective to help you decide how to monetize them. Feel free to contact us to discuss how to make this a commercial success for you.
The authors wish to thank Tommy van Thiel (Senior Consultant) for his contribution to this article.